Table Of Content

If we select a transformation that will shrink the large values more than it does the small values and the overall result would be that we would see less of this fan effect in the residuals. Residuals versus the plot of the fitted values in the upper right-hand corner has now a very distinct pattern. It seems to be a classic as the response gets larger the residuals get more spread apart. The surface plot shows us the same interaction effect in three dimensions in the twisted plane. In addition you can ask Minitab to provide you with 3-D graphical tools that will allow you to grab these boxes and twist them around so that you can look at these boxes in space from different perspectives. These procedures are all 'illustrated in the "Inspect" Flash movie at the beginning of this section.
Questionnaire – Definition, Types, and Examples
As another example, researcher Susan Knasko was interested in how different odors affect people’s behavior [Kna92]. She conducted an experiment in which the independent variable was whether participants were tested in a room with no odor or in one scented with lemon, lavender, or dimethyl sulfide (which has a cabbage-like smell). Although she was primarily interested in how the odors affected people’s creativity, she was also curious about how they affected people’s moods and perceived health—and it was a simple enough matter to measure these dependent variables too. Although she found that creativity was unaffected by the ambient odor, she found that people’s moods were lower in the dimethyl sulfide condition, and that their perceived health was greater in the lemon condition. One of the big advantages of factorial designs is that they allow researchers to look for interactions between independent variables. In this type of study, there are two factors (or independent variables), each with two levels.
Modifying DOE Table
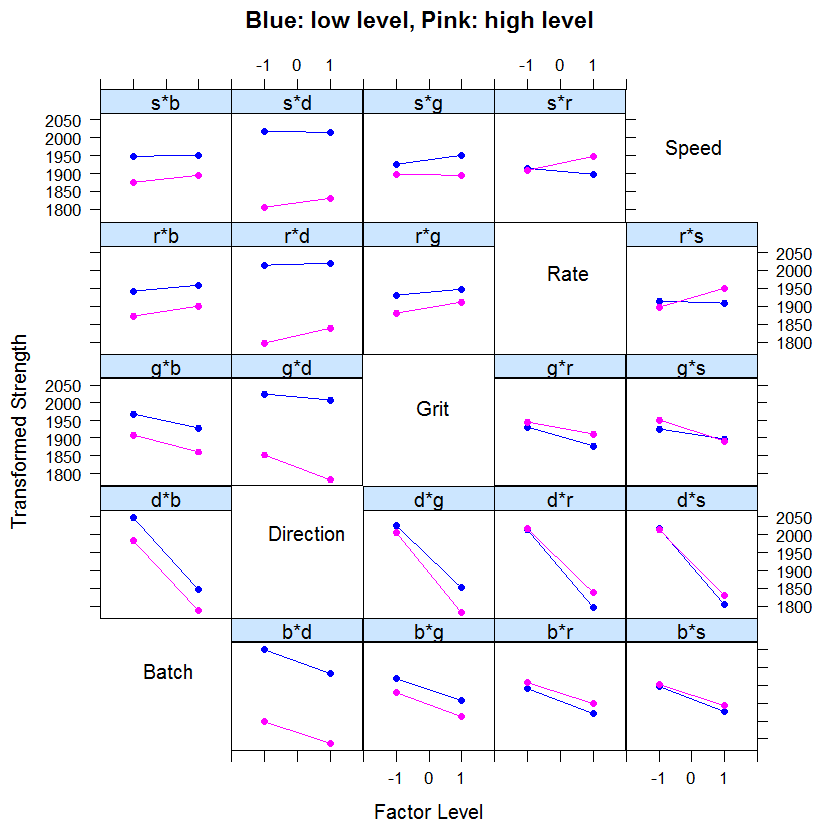
In short, maintaining treatment delivery fidelity may take more care, training and supervision in a factorial experiment than in an RCT. When researchers study relationships among a large number of conceptually similar variables, they often use a complex statistical technique called factor analysis. In essence, factor analysis organizes the variables into a smaller number of clusters, such that they are strongly correlated within each cluster but weakly correlated between clusters. Each cluster is then interpreted as multiple measures of the same underlying construct.
Assigning Participants to Conditions
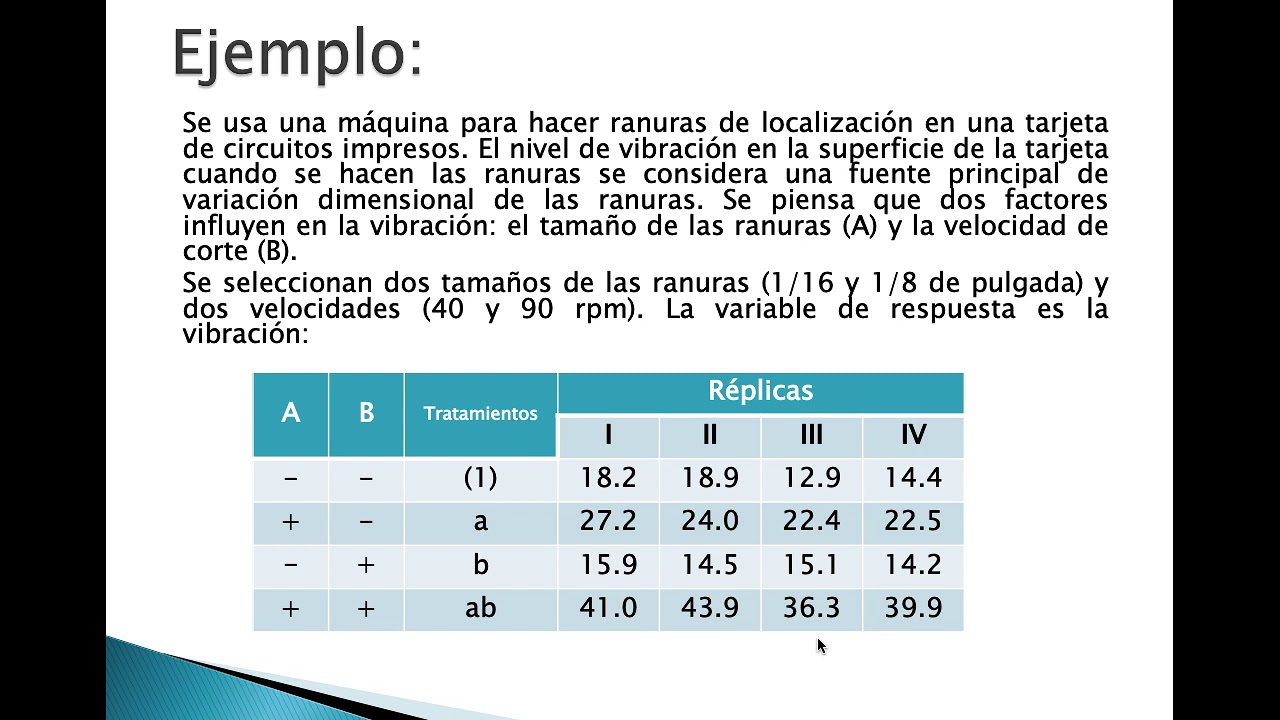
However, the Normal Plot displays whether the effect of the factor is positive or negative on the response. The default factors are named "A", "B", "C", and "D" and have respective high and low levels of 1 and -1. The name of the factors can be changed by simply clicking in the box and typing a new name. Additionally, the low and high levels for each factor can be modified in this menu. Since the high and low levels for each factor may not be known when the design is first created, it is convenient to be able to define them later. A null outcome situation is when the outcome of your experiment is the same regardless of how the levels within your experiment were combined.
Lesson 5: Introduction to Factorial Designs
How design of experiments lowers costs in R&D - Scientific Computing World
How design of experiments lowers costs in R&D.
Posted: Wed, 29 Mar 2023 11:18:22 GMT [source]
Transformations towards the bottom of the list are stronger in how they shrink large values more than they shrink small values that are represented on the plot. This pattern of the residuals is one clue to get you to be thinking about the type of transformations you would select. When we look at the normal probability plot below, created after removing 3-way and 4-way interactions, we can see that now BD and BC are significant.
If you do this, then you simply have a single-factor design, and you are asking whether that single factor caused change in the measurement. For a 2x2 experiment, you do this twice, once for each independent variable. In the table, a yes means that there was statistically significant difference for one of the main effects or interaction, and a no means that there was not a statisically significant difference. As you can see, just by adding one more independent variable, the number of possible outcomes quickly become more complicated. When you conduct a 2x2 design, the task for analysis is to determine which of the 8 possibilites occured, and then explain the patterns for each of the effects that occurred. Again, because neither independent variable in this example was manipulated, it is a non-experimental study rather than an experiment.
You also notice that age does not play a role; both 20 and 40 year olds suffer the same percentage of seizures for a given amount of CureAll. From this information, you can conclude that the chance of a patient suffering a seizure is minimized at lower dosages of the drug (5 mg). The second graph illustrates that with increased drug dosage there is an increased percentage of seizures, while the first graph illustrates that with increased age there is no change in the percentage of seizures. Both of these graphs only contain one main effect, since only dose has an effect the percentage of seizures. Whereas, graphs three and four have two main effects, since dose and age both have an effect on the percentage of seizures. Certainly any research evaluation of intervention effectiveness can pose analytic and interpretive challenges.
V. Chapter 5: Experimental Research
In looking at the interactions, AB, is not significant, BC is not significant, and the ABC are not significant. The quantities b1, b2, and so on are regression weights that indicate how large a contribution an independent variable makes, on average, to the dependent variable. Specifically, they indicate how much the dependent variable changes for each one-unit change in the independent variable. You may find that the patterns of main effects and interaction looks different depending on the visual format of the graph.
5.1. Correlational Studies With Factorial Designs¶
So, for example, a 4×3 factorial design would involve two independent variables with four levels for one IV and three levels for the other IV. Alternatively, an investigator might modify an intervention when it co-occurs with a particular, second intervention component. For instance, assume that a design has three factors; two are medication factors (e.g., varenicline, on/off, in one factor and NRT product [nicotine patch vs. nicotine lozenge], in a second factor). The third factor is an adherence factor (i.e., an automated medication counter with counseling, on/off). Thus, this experiment would address which type of NRT exerts additive or interactive effects when used with varenicline, and whether the adherence intervention exerts main or interactive effects. Obviously the investigator must make the intervention relevant to each medication type, but such adjustment raises questions.
The best they can do is show patterns of relationships that are consistent with some causal interpretations and inconsistent with others. The example in Figure 5.15 shows a case in which it is probably a bit more straightforward to interpret both the main effects and the interaction. Some questions to ask yourself are 1) can you identify the main effect of wearing shoes in the figure, and 2) can you identify the main effet of wearing hats in the figure. Both of these main effects can be seen in the figure, but they aren’t fully clear. To briefly add to the confusion, or perhaps to illustrate why these two concepts can be confusing, we will look at the eight possible outcomes that could occur in a 2x2 factorial experiment. We have usually no knowledge that any one factor will exert its effects independently of all others that can be varied, or that its effects are particularly simply related to variations in these other factors.
In smoking cessation research a common RCT design is one in which participants are randomly assigned to either an active pharmacotherapy or to placebo, with both groups also receiving the same counseling intervention. The vast majority of investigations of treatment efficacy and effectiveness over the past 30–40 years have used the randomized controlled trial (RCT) design. While factorial designs offer some advantages for certain research goals, their use can entail critical decisions regarding design, implementation, analysis, and interpretation. This paper is intended to alert the investigator to such challenges as this may inform decisions about whether to use a factorial design, and how to do so.
It should be quite clear that factorial design can be easily integrated into a chemical engineering application. Many chemical engineers face problems at their jobs when dealing with how to determine the effects of various factors on their outputs. For example, suppose that you have a reactor and want to study the effect of temperature, concentration and pressure on multiple outputs.
The between-subjects design is conceptually simpler, avoids order/carryover effects, and minimizes the time and effort of each participant. The within-subjects design is more efficient for the researcher and controls extraneous participant variables. This would mean that each participant was tested in one and only one condition.
They then submitted these 14 variables to a factor analysis, which identified four distinct factors. These ideas can be confusing if you think that the word “independent” refers to the relationship between independent variables. However, the term “independent variable” refers to the relationship between the manipulated variable and the measured variable. Remember, “independent variables” are manipulated independently from the measured variable. Specifically, the levels of any independent variable do not change because we take measurements. Instead, the experimenter changes the levels of the independent variable and then observes possible changes in the measures.
Because factorial design can lead to a large number of trials, which can become expensive and time-consuming, factorial design is best used for a small number of variables with few states (1 to 3). Factorial design works well when interactions between variables are strong and important and where every variable contributes significantly. If an investigator anticipates severe problems from including a particular factor in an experiment, perhaps due to its nature or the burden entailed, s/he should certainly consider dropping it as an experimental factor. Indeed, the MOST approach to the use of factorial designs holds that such designs be used to decompose a set of compatible ICs, ones that might all fit well in an integrated treatment package (to identify those that are most promising).
No comments:
Post a Comment